In April 2024, BioPharmaTrend published a comprehensive review of the past and current state of artificial intelligence (AI) drug discovery, using the 2012 advent of modern deep learning as a starting point. The review particularly highlighted 9 leading companies in the space that “can design and advance drug candidates de novo (mostly small molecules) and have internal pipelines.”
Given the criticality of patent rights in pharmaceuticals and biotechnology and the impending patent cliff, we analyzed whether trends could be drawn or strategies could be inferred from these 9 exemplary companies’ patent portfolios[1]. This article, part one of two, poses several questions to analyze the companies’ patent strategies and offers high-level conclusions about their portfolios. Part two will dig deeper into how these portfolios are making use of analytics and tactics for effective portfolio development and provide a practical framework to develop and execute on patent strategy in this field.
Analyzing The Patent Portfolios
Here are some key questions to help understand the patent portfolios of the 9 profiled AI drug discovery companies, including how they compare with those of the “big pharma” company portfolios:
- Are these companies protecting aspects of their AI/machine learning (ML) technologies in addition to conventional pharmaceutical technologies e.g., drug compositions, in vivo testing, wet lab screening, etc.?
- Are there any trends over time within AI/ML vs. conventional technologies?
- How well do the patent portfolios map to the publicly known targets that these companies are focused on?
- How do these patent portfolios compare to the total active U.S. patents/pending applications for those same targets?
Below is a first pass evaluation of data[2] that can help answer these questions:
1. Composition vs. AI/ML Protection and Trends over Time
Table 1 provides totals patent assets that can be clearly mapped (based on keywords[3]) to protection for drug compositions or other conventional technologies vs. AI/ML or other computational-first technologies.
Table 1. Filing Landscape (all U.S. patent assets across all companies)
Company | Drug Composition/Conventional Technology Filings (% of total) | AI/ML Filings (% of total) | Total |
Total | 263 (67) | 127 (33) | 390 |
As shown in Table 1, the overall patent filings lean towards conventional pharmaceutical relative to AI/ML (and computational) filings. This may, however, reflect certain companies historically having more conventional filings before expanding their AI/ML presences.
2. Trends Over Time
Figure 1 provides counts of filings since 2012 in each category. Filings for these companies have generally increased in volume for both AI/ML and conventional technologies, with more of the balance towards conventional technologies. These trends may reflect both expansion of the companies’ patenting efforts and the relative new formation of these companies.
Figure 1. Filing Trends by Category
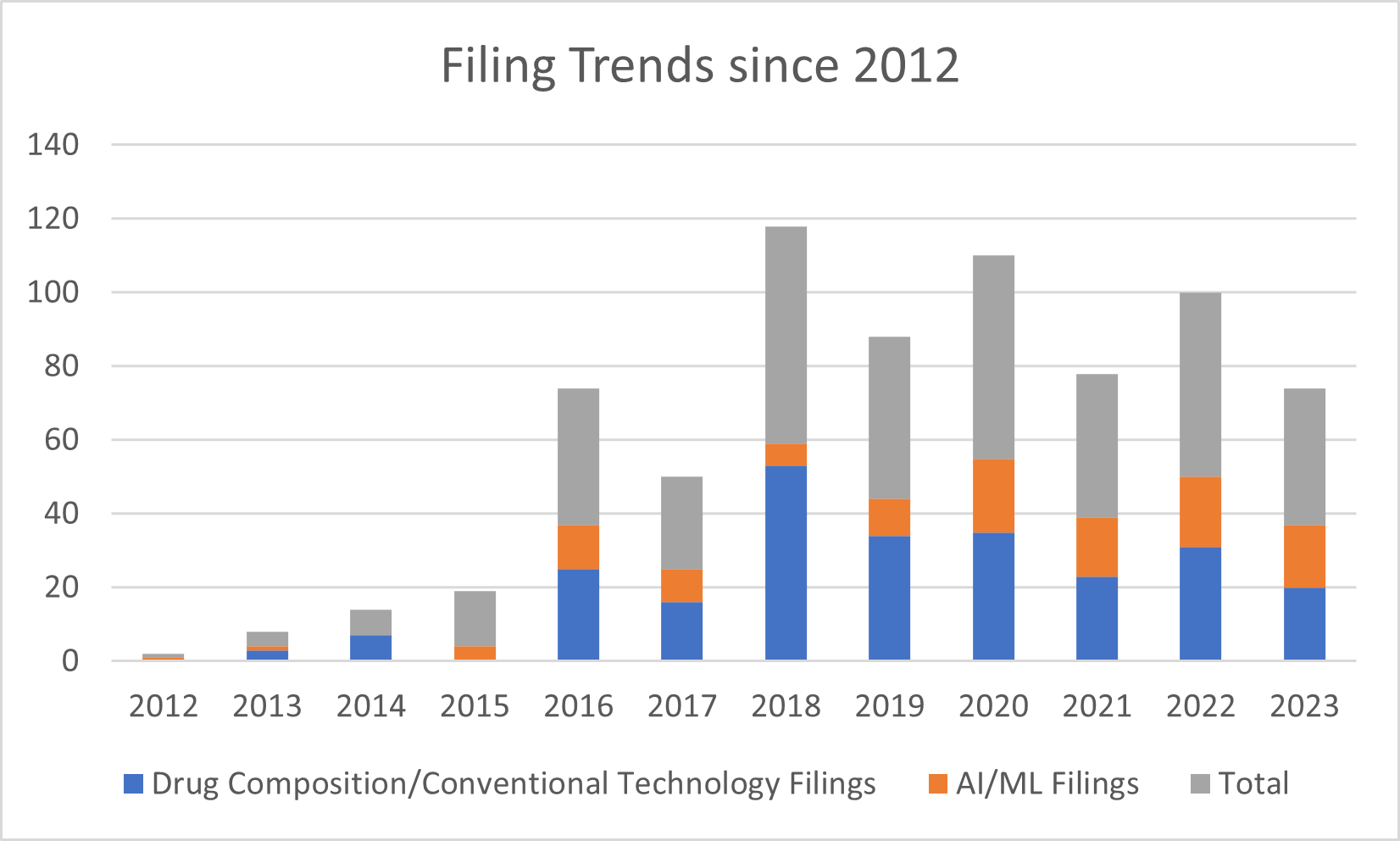
3. Portfolio -> Target Mapping
The hypothesis for these companies is that they would build their patent protection to align with the targets that they have made known to be significant: the most typical patent strategy is to align filings with key commercial priorities. Table 2 below lists the published targets along with a count of patent filings that correspond with those targets, based on keyword searches for the targets in the patent portfolios.[4][5]
Table 2. Target Mapping
Target | Conventional Filings | AI/ML Filings |
---|---|---|
3CLpro | 2 | |
A2aR | 1 | |
APJR | 1 | |
Bcr-Abl | ||
CDC7 | ||
CDC7 | ||
CDK2 | 1 | |
CDK7 | 1 | |
CDKs | 2 | 1 |
CHK1 | ||
cMYC | ||
DGKA | 1 | |
EFGR(C797S) | 10 | |
ENPP1 | ||
ENPP1 | ||
ERα | 8 | |
FGFR2 | 10 | |
FGFR2/3 | 1 | |
HDAC | 1 | |
HIF-2a | ||
HPK1 | ||
HPK1 | ||
HSP90 | 7 | |
JAK2 | 1 | |
KAT6 | ||
LPA1R | ||
LRRK2 | ||
LSD1 | ||
MALT1 | ||
MALT1 | ||
MAT2A | 1 | |
MEK1, MEK2 | ||
Mpro | ||
MYT1 | ||
NLRP3 | ||
NLRP3 | ||
NLRP3 | ||
PARP1 | ||
PARP7 | ||
PDE10 | ||
PHD1/2 | 1 | |
PI3Kα | 10 | |
PIKfyve | ||
PKC, GSK3β | 1 | |
PKC-theta | 1 | |
PRMT5-MTA | ||
QPCTL | ||
RARαβ | ||
RBM39 | 1 | |
ROCK 1/2 inhibitor | ||
S1P1 agonist | ||
SHP2 | 10 | |
SOS1 | ||
TEAD | 1 | |
TNIK | 2 | |
TrkA, TrkB, TrkC | ||
TYK2 | 2 | |
USP1 | 1 | |
USP28 | 5 | |
USP7 | 6 | |
WEE1 | ||
α4β7 | 1 | |
Αvβ8 |
All but two of the AI/ML filings are silent as to their key targets. This leads to several follow-up questions/hypotheses about how these patent portfolios are being developed:
- Does patent protection for AI/ML technologies need to be focused on specific targets? This might depend on how closely the targets are tied to the development of the AI/ML technologies, as opposed to the technologies being generally applicable across targets.
- What other factors drive the selection of the key targets for the AI drug discovery pipeline? The companies may have data moats that are well-integrated with AI/ML technologies, or overall company mission goals tied to specific targets – even if the AI/ML technologies themselves need not be limited to those targets.
- Are patent/IP resources being devoted to goals other than protection directed towards specific targets? On one hand, especially for early-stage companies, it is critical to identify priorities in IP protection and then deploy resources to protect innovations in a manner consistent with these priorities. On the other hand, resource prioritization is all the more reason to focus IP resources on protecting fundamental technologies that tie directly to companies’ commercial priorities.
- Are targets a useful proxy variable to evaluate patent strategy? While targets make it easy to perform a first pass/direct comparison of patent filings and company publications, other factors to consider could include indications, drug classes, or information presented in the patent filings that explain the improvements achieved and what the AI technologies can be used for.
- How does patent publication lag impact forecasting? As noted, the typical 18 month lag from patent filing to publication obscures the most cutting-edge technologies. Looking back at the BioPharmaTrend article, many of the targets identified in Table 1 did not reach move beyond the discovery phase until 2022 or later, and thus may have corresponding patent filings that are not yet public.
4. Comparing AI Company Patent Portfolios with the Overall Landscape
To help provide context for the companies’ patent portfolios, they can also be compared with the overall landscape, with targets as the proxy, to provide an order-of-magnitude assessment of the companies’ share of the overall landscape.
Table 3 lists the approximate total number of U.S. filings for those targets and for the largest filer. Given the sparsity of filings shown in Table 2, this list has been limited to comparing targets where the AI drug discovery companies have at least one filing.
Table 3. Patent Filings by Target Relative to Overall Landscape
Target | Composition | AI/ML | Approximate Total US Filings (Approximate Total for Leading Filer) |
---|---|---|---|
3CLpro | 2 | 3800 (100) | |
A2aR | 1 | 6500 (120) | |
APJR | 1 | 1600 (19) | |
CDK2 | 1 | Total indeterminate (40) | |
CDK7 | 1 | 4000 (100) | |
CDKs | 2 | 1 | Total indeterminate (170) |
DGKA | 1 | 360 (25) | |
EFGR(C797S) | 10 | Total indeterminate (380) | |
ERα | 8 | 10000+ (80) | |
FGFR2 | 10 | Total indeterminate (40) | |
FGFR2/3 | 1 | Total indeterminate (40) | |
HSP90 | 7 | 10000+ (60) | |
JAK2 | 1 | 10000+ (200) | |
MAT2A | 1 | 2800 (15) | |
PHD1/2 | 1 | Total indeterminate (50) | |
PI3Kα | 10 | 2000 (40) | |
PKC, GSK3β | 1 | Indeterminate (180) | |
PKC-theta | 1 | 4000 (40) | |
RBM39 | 1 | 300 (20) | |
S1P1 agonist | Total indeterminate (80) | ||
SHP2 | 10 | 7000 (100) | |
TEAD | 1 | Total indeterminate (10) | |
TNIK | 2 | 400 (50) | |
TYK2 | 2 | 7400 (70) | |
USP1 | 1 | 3000 (60) | |
USP28 | 5 | Total indeterminate (15) | |
USP7 | 6 | Total indeterminate (90) | |
α4β7 | 1 | 3700 (150) |
As seen in Table 3, the total number of filings related to the key targets is typically on the order of hundreds, if not thousands, and the leading filer has tens to hundreds of filings. The discrepancy in volume of filings can be partially explained by the AI drug discovery companies being founded relatively recently. However, this does not change the reality that there are significantly more patents (and possible competitors owning patents) that could be used against emerging AI drug discovery companies than vice versa. Therefore, there may be opportunities left on the table for these companies to develop valuable patents, including (but not limited to) for defensive and/or cross-licensing purposes.
Conclusions
Here are key takeaways from this analysis:
AI drug discovery companies have patent portfolios that are generally balanced between their AI/ML/computational technologies and their drug compositions and other more traditional pharmaceutical/biotechnology inventions. This can reflect several different scenarios that result in similar portfolio makeup: companies that shifted over time from traditional to AI/ML inventions; companies that begin with a balance across the two; and the patent publication lag obscuring more recent filings that might be more AI/ML focused.
These companies may be significantly undervaluing their ability to explicitly protect technologies directed to key targets, especially with the patent cliff coming later this decade. At least on the AI/ML side, this could be because the AI/ML inventions themselves could be broad enough to apply to any target, or may be directed to applications other than target identification and/or drug discovery.
Given the rapid advancement in this space and changes coming in the overall biotechnology/pharmaceutical patent landscape over the next 5-10 years, AI drug discovery companies have a massive opportunity to create strategic advantages with their patent protection. In particular, they can develop protection for drug development across a wide variety of drug and target classes to complement more conventional, composition-focused patents. This protection can prove valuable for direct enforcement against competitors, as well as strategic partnerships and licensing across the biotech/pharma sector.
[1] There is generally an 18-month lag between when patent applications are filed and when they are first published. This can make it challenging to draw strong conclusions about the patent portfolios of early-stage companies and/or companies in rapidly evolving technology areas.
[2] To keep things manageable, these analyses are limited to U.S. patents/applications.
[3] Filings were categorized first based on whether the titles clearly mapped to drug composition or conventional technologies vs. AI/ML technologies and second based on keyword, if necessary. Various cases had overlap between these two categories; some were still categorized as relating to AI/ML even if such technologies focused more on data analysis rather than initial drug design.
[4] See Table 1 in the BioPharmaTrend article; Table 2 here does not delineate amongst targets by phase.
[5] Simply listing a target in a patent filing does not mean a patent resulting from that filing will actually protect the target, but being over-inclusive in this manner provides a first step for contrasting the AI company portfolios with those of more established firms.